Hospital Readmissions and Big Data
Posted on October 16, 2015 |
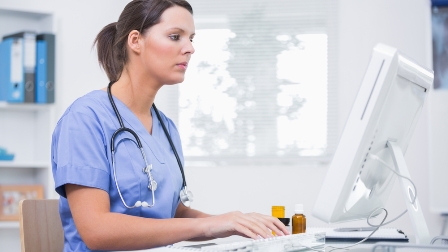
This post was written by Kristen Willard, M.S.
One of the biggest topics in today’s readmissions conversations is data. Big data, predictive analytics, regression analyses – in the context of readmissions, these are all terms that refer to the use of information about patients and your organization that enable professionals and those in their care to make more informed decisions. The approach behind most of these models is to use large data sets to determine readmissions risk and other outcomes for patients with similar clinical and demographic presentations, so that upon admission to the hospital and throughout a stay, providers have an appreciation for 1) a patient’s level of readmissions risk and 2) what measures they can take to maximize a person’s care and prevent a hospital readmission. The primary results? Fewer hospitalizations for patients with COPD and considerable cost savings for the organizations employing these analytic approaches.
Health systems across the country are increasingly using the big data approach (Managed Healthcare Executive article; Intermountain Release. The University of Pittsburgh Medical Center (UPMC) is using analytics in a two-phase process to prevent readmissions. First, a payer-side model determines the patient’s readmission risk based upon predetermined risk factors. Second, a provider-side model uses lab and clinical data extracted from the medical record to provide real-time updates on patient status and readmissions risk. Two models working in tandem can be challenging, the organization notes, but ultimately the organization has adopted a system that works for them and has equipped the team to make considerable cuts in their system’s readmissions rates.
Now Atrium Health, Carolinas Healthcare System (CHS) adopted their own predictive analytics approach that assisted them in reducing COPD readmissions rates. Working with Predixion Software, they built a model that classified patients into very high, high, medium and low risk categories for 30-day readmissions. Care teams led by case managers could then examine individual patient risk records at the point of care and determine which comorbidities or other factors might impact their readmissions risk and personalize their interventions accordingly. The approach enabled CHS to reduce the readmissions rate by 7 percentage points over two years. (See the PRAXIS Resource Repository.)
As with the implementation of any new approach, there are challenges to the use of data sets in our efforts to improve care. First, the authors assert, having access to large sets of raw information does not mean they translate to usable outcomes. Second, “insight and value are not the same”; that is, there are many scientific results which may be statistically significant but fall short when considering their real-world clinical significance. Third, one must consider the quality of the data in question and what inferences can be drawn from the results. The authors encourage us to think of the example of weather forecasting; while the data are high quality and the approaches considered precise, even the best prediction models result in inaccurate calls. Last, both implementing an analytics approach and the interventions suggested by its results may require significant cultural change and resources on behalf of organizations and their stakeholders.
Additional articles of interest can be found here:
Encompass Health via Modern Healthcare; Healthcare Finance News. Journal articles of interest include A predictive analytics approach to reducing 30-day avoidable readmissions among patients with heart failure, acute myocardial infarction, pneumonia, or COPD as well as an earlier article from Predicting Hospital Readmission Risk for COPD Using EHR Information.
What are your thoughts on predictive analytics and big data? If you've used these in your work, where did you start?
This page was reviewed on March 6, 2020 by the COPD Foundation Content Review and Evaluation Committee